

A Recent History of Camera Platforms, and Future Predictions
We cannot deny that almost everyone is a photographer these days. In 2022, smartphone cameras have become so ubiquitous, and make it so easy and fun to take decent snapshots, that many of us have forgotten the age of film or even compact cameras from a decade or so ago.
And now, if we compare the quality of the shots from those point-and-shoot cameras, or the images on the first camera phones to what we capture out of the box by a modern smartphone, the differences are astounding.
However, despite these differences, there still exists a gap between today’s flagship smartphone cameras and the image quality achievable in a modern standalone DSLR (Digital Single Lens Reflex) or mirrorless digital camera.
Let’s look at some of the reasons for the improvements that have happened, the reasons for the quality gap that remains, and what the technological trends suggest will happen in the future.
There is also good reason to believe that the easy gains in smartphone image quality have already been made. We’ll elaborate on why this is the case and what could be done to help them catch up with the best professional DSLR cameras.
First, let’s discuss some of the pros and cons of these different camera platforms, and why photo quality matters.
Camera Quality vs.Convenience: Preserving Our Best Memories
DSLRs are heavy, bulky, and inconvenient - so much so that even those of us who own them tend to leave them at home unless it’s for a special event. Also, they often have poorly thought out user interfaces for the casual user, making them hard to master.
In contrast, it’s easy to take a quick snap with the phones that we all carry with us everywhere. We can bet that an image will come out well exposed and focused. But is that all you need for a good shot?
QUALITY IS IMPORTANT. So many of us capture memories from important points in our lives that we hope to preserve for years to come. For example, at special events, weddings, reunions, vacations, and of the kids growing up. These are times that we will want to look back on and cherish later.
Imagine in ten years from now looking at a grainy, blurry, murky image taken at night and wishing it was preserved in greater fidelity. If only the camera had been better, we will muse.
Smartphones are great for quick snaps, and when viewed on the phone’s screen itself, they usually look great. But pinch and zoom in, and often the details look murky, grainy, crunchy, or plastic and overly processed.
Show it on a large-screen 8k TV, try to make a big print for the wall, or even download to a computer and crop, and the details just are not there.
And that’s just today’s technologies - imagine viewing on AR/VR headsets in the years to come that will have lifelike clarity. Images may look good at first glance on the phone now, but compare them side by side with the same scene shot with a “proper” standalone camera, and you will find that something is lacking.

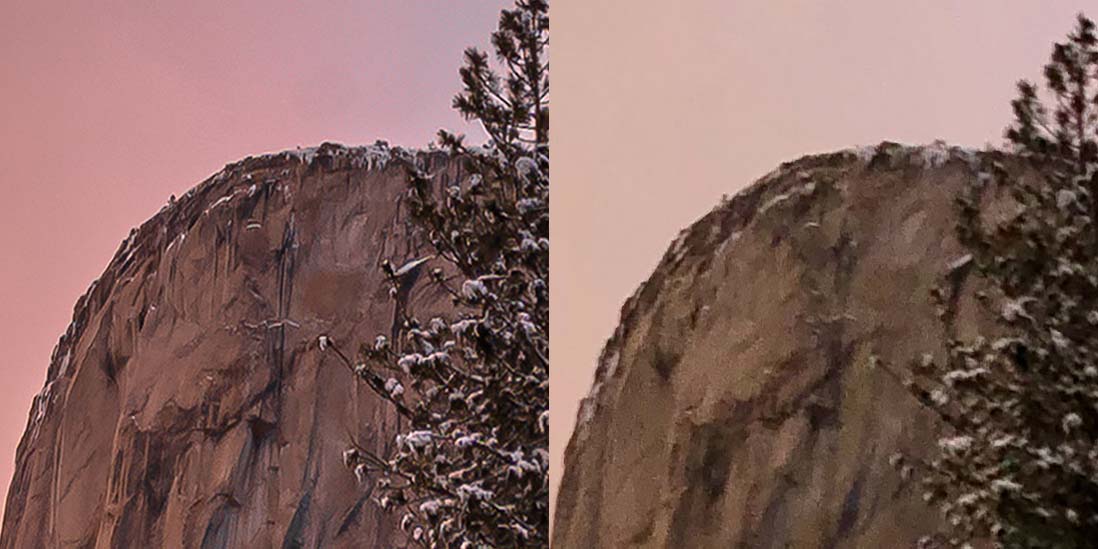
Manufacturers may advertise increasing MegaPixel resolutions; however, those numbers don’t reflect the entire truth about quality.
Capture the Light: Better Camera Hardware
Fundamentally, cameras capture photons of light to form an image on a digital sensor (or film). If there isn’t enough light, the resulting image will tend to be grainy and noisy, or blurred from a long exposure time.
Sensor resolution (Megapixel count) is often touted as a headline hardware spec. However, it is typically less important than the following 4 main technical factors that dramatically affect quality from a hardware and physics perspective:
- Optical light-gathering power: Size of the lens / aperture
- Electronic light-gathering power: Area of the sensor
- Exposure time
- Sensitivity of the sensor (silicon efficiency, pixel, and microlens design)
Smartphones are fundamentally constrained to be thin devices that should fit in our pockets.
This limits both of the first two factors: the lens and sensor must be small, based on the geometry of the smartphone’s design.

Still, smartphones have pushed this envelope to the limit by reversing the trend of a few years ago to get thinner; instead “camera bumps” started to appear. These allow a physically bigger camera to protrude from a thin phone.
That trend will be hard to extend any further for product design and practical usability reasons. DSLRs or mirrorless cameras have APS-C or full-frame sensors that are often 10 to 100 times larger in area, giving them a huge advantage in this regard.
Larger apertures (lower f/numbers) have slowly emerged as more real estate is given up to cameras, and pixel designs and manufacturing techniques have enabled these to reach near their optimum levels.
Smartphones have also added multiple cameras, enabling capture optimized for different focal lengths (zoom factors). However, this adds complexity and additional power requirements, so there is always a tradeoff in the race to add more and more lenses.
The third factor above (exposure time) is limited by the steadiness of the camera and by motion in the scene.
Using a tripod allows for a longer exposure, but this is hardly convenient for most shots. Instead, the development of Optical Image Stabilization (OIS) into sensors or lenses to counteract hand motion allows more light to be integrated during the exposure.
The fourth factor (sensitivity) has somewhat improved over the last decade or two, as better silicon manufacturing processes have enabled more of the photons hitting the sensor to be turned into electrical charge signals that form the digital image.
Technologies such as Back Side Illumination (BSI), better analog-to-digital circuitry, improved color filter arrays, and optimized microlenses capture more of the incident light rays.
Yet all these sensor improvements have also been applied to large DSLR / mirrorless digital cameras. So, what has enabled smartphone image quality to get so much better?
Photography Software to the Rescue
The main reason for improved quality in smartphones is the exponential growth in the processing power available on these platforms, and consequently the much improved software algorithms that are possible. These algorithms come from the realm of computer vision and computational imaging, and more recently, advanced AI using neural networks.
Smartphones have become so powerful that they eclipse the processing power of desktop computers from a few years ago. This is due to their ubiquity and popularity, and therefore the immense resources that have been poured into designing custom chips dedicated to tasks such as photo and video processing as well as machine learning and AI.
At the same time, advanced research has improved the software algorithms that run on these chips using noise reduction and clarity-enhancing techniques to bring out more detail in exactly the same image file.
Comparing a RAW image (close to the signal captured by the sensor) processed into a JPEG file using on-camera software from around a decade ago to the same RAW image but processed by the latest AI-based algorithms found on both recent smartphone Image Signal Processor (ISP) chips, or consumer RAW processing software, the difference can be astounding.

In theory, DSLRs can take advantage of the same improvements in processing on camera. However, they typically lag a few years behind in terms of using the latest chips and processing techniques. This is because of their smaller market share and the reluctance of traditional camera makers to move as quickly as phone companies.
Let’s take a look at some of the particular software algorithms, often emerging from the world of computational photography, which have improved smartphone images so much in recent years.
Examples of Software-Based Computational Image Processing
Denoising and Sharpening
One of the main issues mentioned above, when limited amounts of light are captured, is image noise. It appears as random patterns, or like “static” overlaid on the image.
Applied to a single frame, spatial image noise reduction methods use statistical techniques to average out the noise while preserving the useful signal of the image, in the form of edge and texture detail.

Sharpening is also applied as a separate or combined process to try to emphasize these details while avoiding boosting the noise patterns too much.
Early algorithms usually gave an overly smooth, plastic, or cartoonish look with harsh over-sharpened edges and suppressed fine detail. In recent years, more advanced methods have delivered remarkable gains with a higher computational burden, by analyzing larger neighborhoods of the image to distinguish subtle features better.
Deep Neural Networks (DNNs) take this to the next level, training on large datasets of noisy and clean images and automatically learning a mapping from the former to the latter.
HDR And Tone Mapping
The small pixel sizes typically used in smartphones of around 0.7-1.5 microns result in a small well capacity for electrons, meaning a limited dynamic range. This means that a single shot will struggle to expose properly to capture detail in bright areas (which are blown out) and shadows (which are lost to noise), particularly in backlit scenes.
By capturing multiple frames with different exposure times and registering (aligning) them, both highlights and shadows may be preserved. These High Dynamic Range (HDR) images may then be tone mapped to the visible display range. This gives a pleasing visual effect that boosts local shadow detail while preserving highlights.
Multi-frame Fusion (Night Mode, Deep Fusion, Night Sight, etc.)
In modern sensors, photon shot noise typically dominates over dark current noise. This has enabled bursts of short-exposure photos to be used and combined into a single high-quality image, for the purpose of noise reduction and stabilized long exposure imaging (in addition to HDR).
These frames are aligned automatically (globally or locally) to compensate for hand movement, while scene movement, which would otherwise cause ghosting artifacts, is detected and suppressed.
Other variants use combinations of short and long photos to enable “seeing in the dark” in low-light situations. Again, AI techniques using DNNs can improve the quality of these types of results, often with a heavier computational burden.
Super-resolution
The desire to provide additional zoom, cropping, or resolution from low-resolution sensors has led to the development of Super-resolution (SR) techniques. These may be single image SR, which is essentially a form of smart upsampling, or multi-frame methods.
Single image approaches mainly require the use of machine learning to achieve their results, training on patches of low and high-resolution images to learn the typical structure of fine image details.
In doing so, they statistically recreate likely patterns that are probable to occur. However, in essence, they are inventing details as a painter would since the true detail is lost in the sampling process on the sensor. These techniques may also account for blur or softness induced by the lens.
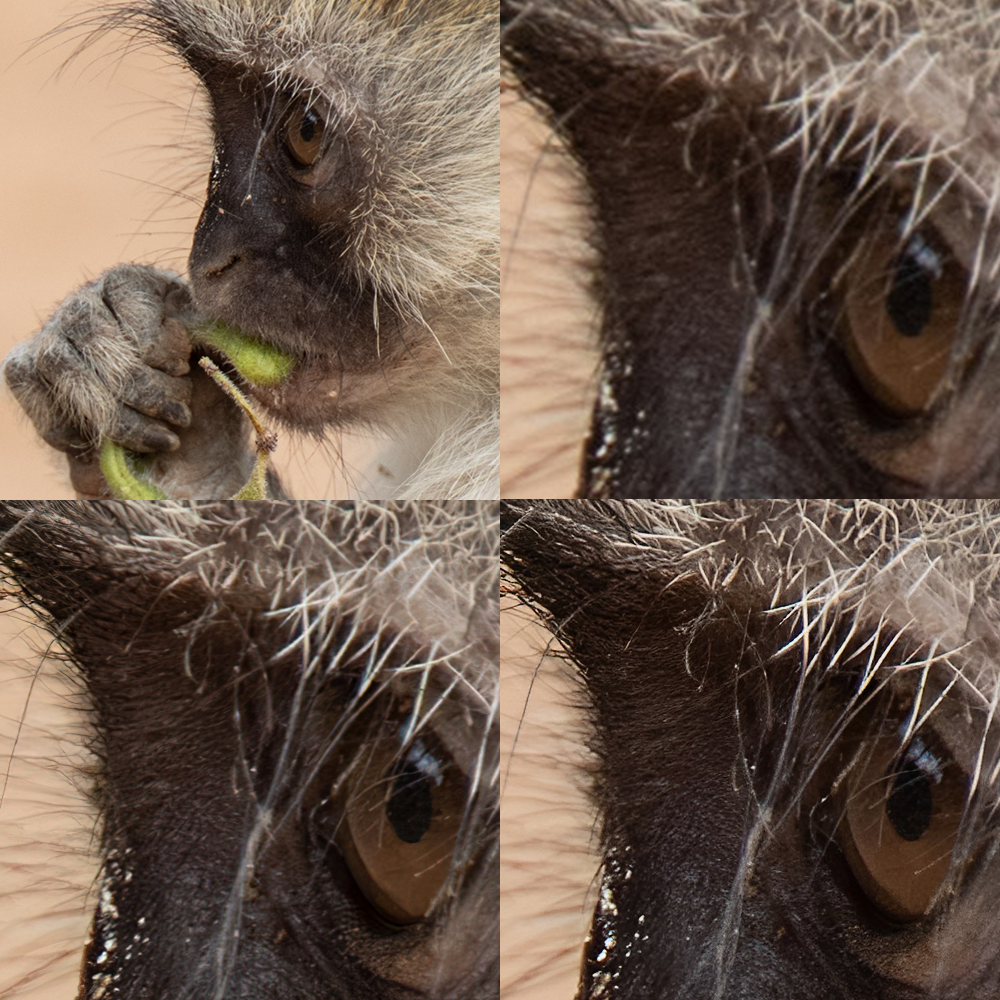
Multi-frame SR approaches must also account for this blurring effect and assume that there is an amount of aliasing (under-sampling as in a moiré pattern) present. Unlike single-frame methods, this assumes that details are preserved and distributed between the frames but just need to be recovered.
A variation on this approach is RAW-based SR, which accounts for the under-sampling present in the Bayer pattern or Color Filter Array (CFA) that is used on the sensor. This can give dramatic increases in detail for color images when the alignment between shots is well calibrated.
Shallow Depth of Field, aka Portrait Mode
The previous techniques focused on quality in terms of detail, noise, and dynamic range, which are all important for giving a good local appearance to the image.
Aside from this, one major difference between large and small cameras is their depth of field. This refers to the portion of the scene in focus: small aperture cameras, such as those found on smartphones tend to render everything in focus at once.
Larger format DSLR/mirrorless cameras can focus on a single plane, which is a classic look for portrait photography; the background is rendered smooth and out of focus, helping to isolate the subject.
In recent years, software has enabled the recreation of this effect by firstly using depth estimation methods to predict the distance to objects in the scene and then rendering an artificial blur in these areas according to the depth.
The out-of-focus highlights are termed “Bokeh” by photographers, and the recreation of good Bokeh is often deemed one characteristic of a good lens. Recent efforts are moving towards simulating these looks more and more accurately.
However, the prediction of accurate depth or segmentation of the image is challenging. Typical techniques involve either stereo cameras, focus pixels, structured light, Time-of-Flight (ToF) LIDAR sensors, or machine learning algorithms.
These methods have improved over time, but are still not perfect when segmenting fine edge details, such as hair, or with foreground areas that have similar color as the background.
The result is that synthetic Bokeh or portrait mode type methods may have rendering artifacts. And while they are still compelling, there is still a significant gap between the effect from a smartphone and what a real shallow depth of field image from a larger camera looks like.
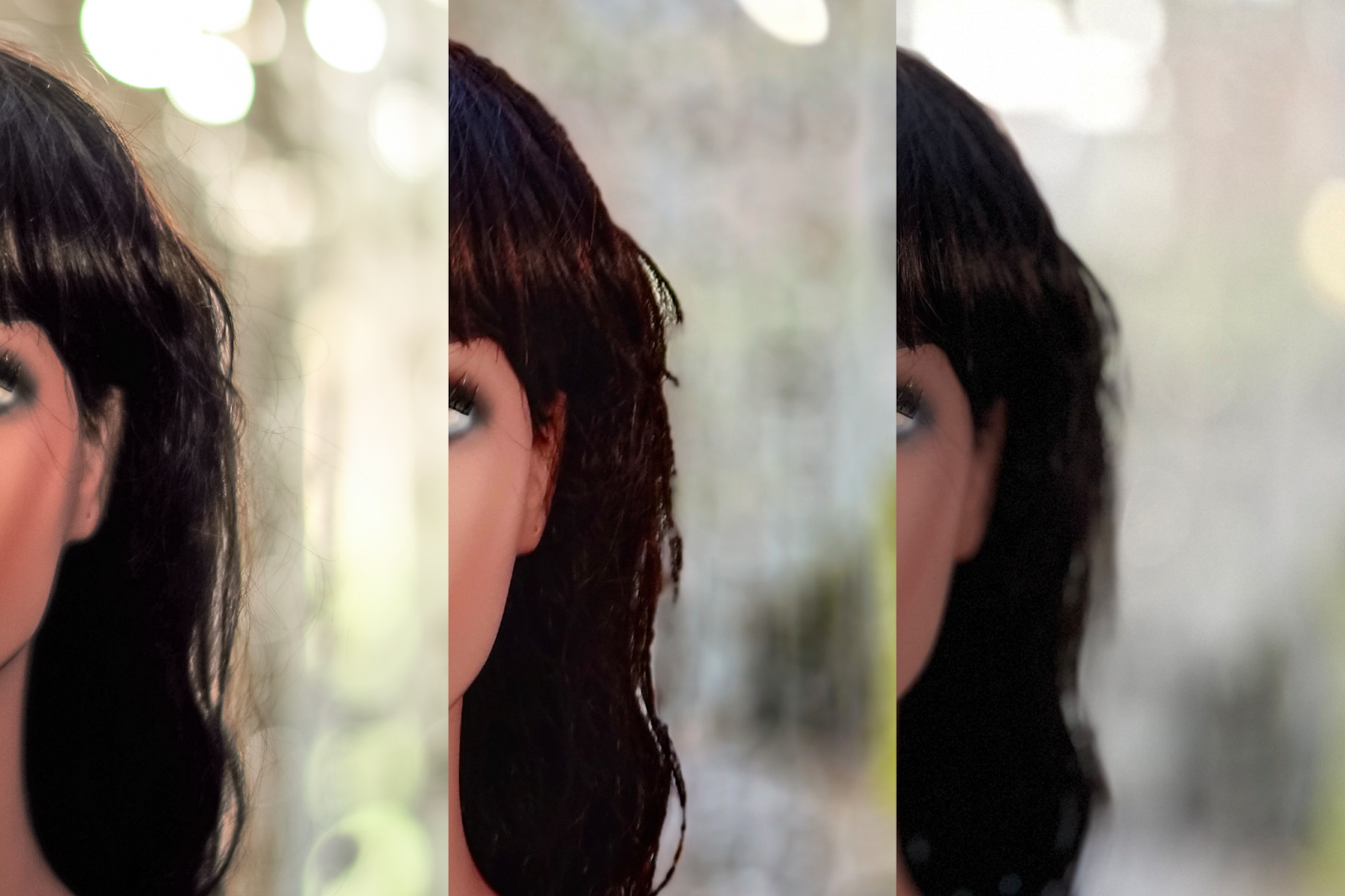
Algorithmic Acceleration
As all these algorithms mature, they typically transition from running on CPU or GPU to being embedded in custom silicon (ASICs) as part of the ISP. However, these days, as more parts of the imaging pipeline are running as Deep Neural Networks (DNNs), they can run easily on the powerful Neural Engines that are part of most modern smartphones.
Increasingly many of these steps are being combined into a single end-to-end model that can be trained using machine learning.
DSLRs, by comparison, tend to use custom silicon that is years out of date compared to smartphones and are typically unable to run these algorithms as efficiently. It is likely that they will eventually catch up by including DNN based engines in their silicon, which should see another boost in their quality.
What Comes Next for the Smartphone Camera?
As we have discussed, hardware improvements in the last decade have captured most of the easy gains, and these have been applied to both large and small cameras. As a result, they have not provided a particularly outsized gain in smartphones over DSLRs / mirrorless cameras.
Computational imaging techniques, including multi-shot fusion and advanced noise reduction methods, have delivered outside improvements for smartphones. However, these have now reached maturity in the sense that most of the potential for post-processing techniques has now been delivered. This is because the powerful AI engines in today’s phones handle the most complex algorithms that were previously too heavy to deploy in a portable computing device.
Most of these methods have assumed that the software image restoration techniques are applied post-hoc, i.e., to address various limitations of the hardware or physics of the optical imaging system.
A further stage in theoretical computational imaging design that we believe will become prevalent in the industry in the near future is the joint design of optical hardware and software processing methods.
This joint development can allow for novel optical designs that bend the rules of traditional camera and lens principles, with the assumption that the software and hardware work hand in hand to deliver an exceptional image.